Healx scientist publishes paper predicting effectiveness of combinations of antibiotics
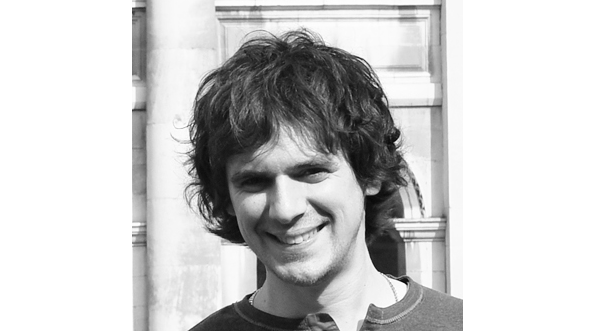
Daniel Mason, Molecular Informatics Data Scientist at Healx, has developed a machine learning approach to predict the effectiveness of combinations of antibiotics, potentially reducing the time and resources spent on experimental screening for new treatments. Such an approach also shows potential for repurposing approved drugs for novel combination treatments.
Daniel developed the tool, called Combination Synergy Estimation (CoSynE), during his postdoctoral research associate position at the University of Cambridge. CoSynE uses compound combination experimental screening data, together with knowledge of the structure of compounds, to predict whether or not a novel combination is likely to exert a synergistic effect, that is, activity greater than the sum of its parts. These predictions can then be used to prioritise the testing of new combinations in subsequent assays.
The approach has also been used with a number of other experimental combination datasets, and is being investigated for use in other areas such as drug repurposing.
Daniel worked under the supervision of Dr Andreas Bender, Chief Technology Officer at Healx. Dr Bender is a Reader for Molecular Informatics with the Centre for Molecular Science Informatics at the Department of Chemistry of the University of Cambridge.
The work described here is published in the the Journal of Medicinal Chemistry.